This will likely be a multipart post going over everything required to get caffe running on Windows with python bindings for use in ipython notebooks and jupyter.
Preliminaries
Build environment:Windows 10 x64
MSVC 2015 Community. Update 0 <- This is important, cuda doesn't work against MSVC 2015 2 and greater.
I will be installing all libraries to: G:/libs
I will be building source code in: G:/code
I use tortoiseGit for my git ui.
I will be building against the following libraries:
Python 3.5
- Installed to C:/python35
Boost 1.61 (Compiled from source against Python 3.5)
- Installed to G:/libs/boost_1_61_0
OpenCV 3.1 from git
- Installed to G:/libs/opencv
CUDA 8.0
- Installed to default program files location
Other dependencies
- Cloned to G:/libs/caffe_deps
Optional:
Qt 5.7
- Installed to C:/qt/5.7/msvc2015_64
VTK 7.1
- Installed to G:/libs/vtk
GStreamer 1.8.2 (1.9.2 has a linking bug that you wont run into until linking OpenCV)
- Installed to G:/libs/gstreamer
Python 3.5
Python was installed from binary
Boost
Boost was compiled from source, it automatically picked up the python libraries and includes, however if it does not automatically pick up python, you can manually set the following settings in your project-config.jam file.
using python : # version : c:/Python35 # cmd-or-prefix : C:/Python35/include : C:/python35/libs/python35.lib ;
If you download the binary distribution, boost will be compiled against Python 2.7.
Boost from source: BOOST_LIBRARYDIR=G:/libs/boost_1_61_0/stage/lib
Boost from binary: BOOST_LIBRARYDIR=G:/libs/boost_1_61_0/lib64-msvc-14.0
OpenCV
Right click the code folder and click clone.
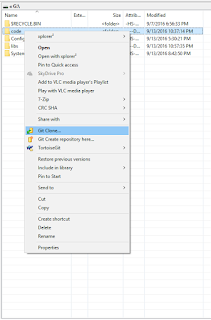
Enter the url for OpenCV's repository, then click OK. This will checkout the master branch from OpenCV's git.
Thrust bug fix in CUDA 8.0 RC
Thrust has a bug that prevents OpenCV from compiling correctly, the fix is to checkout the newest thrust from git and overwrite the one installed with CUDA 8.0.
Following the same procedure, clone http://github.com/thrust/thrust
Inside the newly created thrust folder "G:/code/thrust" for me, copy the thrust (G:/code/thrust/thrust) folder into C:\Program Files\NVIDIA GPU Computing Toolkit\CUDA\v8.0\include, overwriting the one that currently exists in that folder. The include files should match up such that you're overwriting mostly the same files.
OpenCV CMake settings:
General configuration for OpenCV 3.1.0-dev =====================================
Version control: 2.4.9-10234-gc3b8b36-dirty
Platform:
Timestamp: 2016-09-16T02:12:34Z
Host: Windows 10.0.10240 AMD64
CMake: 3.5.0-rc1
CMake generator: Visual Studio 14 2015 Win64
CMake build tool: C:/Program Files (x86)/MSBuild/14.0/bin/MSBuild.exe
MSVC: 1900
C/C++:
Built as dynamic libs?: YES
C++ Compiler: C:/Program Files (x86)/Microsoft Visual Studio 14.0/VC/bin/x86_amd64/cl.exe (ver 19.0.23026.0)
C++ flags (Release): /DWIN32 /D_WINDOWS /W4 /GR /EHa /D _CRT_SECURE_NO_DEPRECATE /D _CRT_NONSTDC_NO_DEPRECATE /D _SCL_SECURE_NO_WARNINGS /Gy /bigobj /Oi /wd4251 /wd4324 /wd4275 /wd4589 /MP24 /openmp /MD /O2 /Ob2 /D NDEBUG /Oy- /Zi
C++ flags (Debug): /DWIN32 /D_WINDOWS /W4 /GR /EHa /D _CRT_SECURE_NO_DEPRECATE /D _CRT_NONSTDC_NO_DEPRECATE /D _SCL_SECURE_NO_WARNINGS /Gy /bigobj /Oi /wd4251 /wd4324 /wd4275 /wd4589 /MP24 /openmp /D_DEBUG /MDd /Zi /Ob0 /Od /RTC1
C Compiler: C:/Program Files (x86)/Microsoft Visual Studio 14.0/VC/bin/x86_amd64/cl.exe
C flags (Release): /DWIN32 /D_WINDOWS /W3 /D _CRT_SECURE_NO_DEPRECATE /D _CRT_NONSTDC_NO_DEPRECATE /D _SCL_SECURE_NO_WARNINGS /Gy /bigobj /Oi /MP24 /openmp /MD /O2 /Ob2 /D NDEBUG /Zi
C flags (Debug): /DWIN32 /D_WINDOWS /W3 /D _CRT_SECURE_NO_DEPRECATE /D _CRT_NONSTDC_NO_DEPRECATE /D _SCL_SECURE_NO_WARNINGS /Gy /bigobj /Oi /MP24 /openmp /D_DEBUG /MDd /Zi /Ob0 /Od /RTC1
Linker flags (Release): /machine:x64 /INCREMENTAL:NO /debug
Linker flags (Debug): /machine:x64 /debug /INCREMENTAL
Precompiled headers: YES
Extra dependencies: comctl32 gdi32 ole32 setupapi ws2_32 Qt5::Core Qt5::Gui Qt5::Widgets Qt5::Test Qt5::Concurrent Qt5::OpenGL vfw32 G:/libs/gstreamer/1.0/x86_64/lib/gstaudio-1.0.lib G:/libs/gstreamer/1.0/x86_64/lib/gstbase-1.0.lib G:/libs/gstreamer/1.0/x86_64/lib/gstcontroller-1.0.lib G:/libs/gstreamer/1.0/x86_64/lib/gstnet-1.0.lib G:/libs/gstreamer/1.0/x86_64/lib/gstpbutils-1.0.lib G:/libs/gstreamer/1.0/x86_64/lib/gstreamer-1.0.lib G:/libs/gstreamer/1.0/x86_64/lib/gstriff-1.0.lib G:/libs/gstreamer/1.0/x86_64/lib/gstrtp-1.0.lib G:/libs/gstreamer/1.0/x86_64/lib/gstrtsp-1.0.lib G:/libs/gstreamer/1.0/x86_64/lib/gstsdp-1.0.lib G:/libs/gstreamer/1.0/x86_64/lib/gsttag-1.0.lib G:/libs/gstreamer/1.0/x86_64/lib/gstvideo-1.0.lib G:/libs/gstreamer/1.0/x86_64/lib/glib-2.0.lib G:/libs/gstreamer/1.0/x86_64/lib/gstapp-1.0.lib G:/libs/gstreamer/1.0/x86_64/lib/gobject-2.0.lib vtkRenderingOpenGL vtkImagingHybrid vtkIOImage vtkCommonDataModel vtkCommonMath vtkCommonCore vtksys vtkCommonMisc vtkCommonSystem vtkCommonTransforms vtkCommonExecutionModel vtkDICOMParser vtkIOCore vtkzlib vtkmetaio vtkjpeg vtkpng vtktiff vtkImagingCore vtkRenderingCore vtkCommonColor vtkFiltersGeometry vtkFiltersCore vtkFiltersSources vtkCommonComputationalGeometry vtkFiltersGeneral vtkInteractionStyle vtkFiltersExtraction vtkFiltersStatistics vtkImagingFourier vtkalglib vtkRenderingLOD vtkFiltersModeling vtkIOPLY vtkIOGeometry vtkFiltersTexture vtkRenderingFreeType vtkfreetype vtkIOExport vtkRenderingGL2PS vtkRenderingContextOpenGL vtkRenderingContext2D vtkgl2ps glu32 opengl32 cudart nppc nppi npps cublas cufft -LIBPATH:C:/Program Files/NVIDIA GPU Computing Toolkit/CUDA/v8.0/lib/x64
3rdparty dependencies: zlib libjpeg libwebp libpng libtiff libjasper IlmImf
OpenCV modules:
To be built: cudev core cudaarithm flann imgproc ml video viz cudabgsegm cudafilters cudaimgproc cudawarping imgcodecs photo shape videoio cudacodec highgui objdetect ts features2d calib3d cudafeatures2d cudalegacy cudaobjdetect cudaoptflow cudastereo stitching superres videostab
Disabled: world
Disabled by dependency: -
Unavailable: java python2 python3
Windows RT support: NO
GUI:
QT 5.x: YES (ver 5.7.0)
QT OpenGL support: YES (Qt5::OpenGL 5.7.0)
OpenGL support: YES (glu32 opengl32)
VTK support: YES (ver 7.1.0)
Media I/O:
ZLib: build (ver 1.2.8)
JPEG: build (ver 90)
WEBP: build (ver 0.3.1)
PNG: build (ver 1.6.24)
TIFF: build (ver 42 - 4.0.2)
JPEG 2000: build (ver 1.900.1)
OpenEXR: build (ver 1.7.1)
GDAL: NO
GDCM: NO
Video I/O:
Video for Windows: YES
DC1394 1.x: NO
DC1394 2.x: NO
FFMPEG: YES (prebuilt binaries)
codec: YES (ver 57.48.101)
format: YES (ver 57.41.100)
util: YES (ver 55.28.100)
swscale: YES (ver 4.1.100)
resample: NO
gentoo-style: YES
GStreamer:
base: YES (ver 1.0)
video: YES (ver 1.0)
app: YES (ver 1.0)
riff: YES (ver 1.0)
pbutils: YES (ver 1.0)
OpenNI: NO
OpenNI PrimeSensor Modules: NO
OpenNI2: NO
PvAPI: NO
GigEVisionSDK: NO
DirectShow: YES
Media Foundation: NO
XIMEA: NO
Intel PerC: NO
Parallel framework: OpenMP
Other third-party libraries:
Use IPP: 9.0.1 [9.0.1]
at: G:/code/opencv/3rdparty/ippicv/unpack/ippicv_win
Use IPP Async: NO
Use Lapack: NO
Use Eigen: YES (ver 3.2.9)
Use Cuda: YES (ver 8.0)
Use OpenCL: YES
Use OpenVX: NO
Use custom HAL: NO
NVIDIA CUDA
Use CUFFT: YES
Use CUBLAS: YES
USE NVCUVID: NO
NVIDIA GPU arch: 20 30 35 50 60
NVIDIA PTX archs:
Use fast math: NO
OpenCL: <Dynamic loading of OpenCL library>
Include path: G:/code/opencv/3rdparty/include/opencl/1.2
Use AMDFFT: NO
Use AMDBLAS: NO
Python 2:
Interpreter: C:/Python35/python.exe (ver 3.5)
Python 3:
Interpreter: C:/Python35/python.exe (ver 3.5)
Python (for build): C:/Python35/python.exe
Java:
ant: NO
JNI: NO
Java wrappers: NO
Java tests: NO
Matlab: Matlab not found or implicitly disabled
Documentation:
Doxygen: NO
PlantUML: NO
Tests and samples:
Tests: YES
Performance tests: YES
C/C++ Examples: NO
Install path: G:/libs/opencv
cvconfig.h is in: G:/code/opencv/build
-----------------------------------------------------------------
So basically the procedure to get OpenCV working is:
Set the GSTREAMER_DIR path variable to G:/libs/gstreamer/1.0/x86_64:
Set the VTK_DIR path variable to G:/libs/vtk/lib/cmake/vtk-7.1
Set the following to true:
WITH_QT
WITH_CUDA
WITH_CUBLAS
WITH_VTK
WITH_OPENGL
WITH_OPENMP
Hit configure, upon setting these variables several new variables should pop up.
Set the following QT variables as needed:
Qt5Concurrent_DIR = C:/Qt/5.7/msvc2015_64/lib/cmake/Qt5Concurrent
Qt5Core_DIR = C:/Qt/5.7/msvc2015_64/lib/cmake/Qt5Core
Qt5Gui_DIR = C:/Qt/5.7/msvc2015_64/lib/cmake/Qt5Gui
Qt5OpenGL_DIR = C:/Qt/5.7/msvc2015_64/lib/cmake/Qt5OpenGL
Qt5Test_DIR = C:/Qt/5.7/msvc2015_64/lib/cmake/Qt5Test
Qt5Widgets_DIR = C:/Qt/5.7/msvc2015_64/lib/cmake/Qt5Widgets
(optional) Set the following extra variables:
CMAKE_INSTALL_PREFIX=G:/libs/opencv
Hit Generate to create the visual studio project.
Open the project and build everything, once everything is built, run the INSTALL project in the CMakeTargets folder.
Building Caffe
At this point we should have OpenCV 3.1 compiled against our desired flavor of Python, CUDA 8.0, and a few other goodies. We should also have Boost 1.61 with the Python bindings for our desired python version. To test this, load up python and type:
import cv2
If this fails, it is likely due to missing dependent DLLs that need to be in your path. To fix this, just add the following to your path:
G:/libs/vtk/bin
G:/libs/opencv/x64/vc14/bin
${BOOST_LIBRARYDIR}
G:/libs/gstreamer/1.0/x86_64/bin
Now time for setting up Caffe.
Clone my fork of the repo:
Checkout the "merge" branch.
Clone caffe_deps into G:/libs/caffe_deps.
Checkout the VS2015 branch.
Caffe cmake config
Manually add the following:
BOOST_ROOT=G:/libs/boost_1_61_0
Boost from source:
BOOST_LIBRARYDIR=G:/libs/boost_1_61_0/stage/lib
Boost from binary:
BOOST_LIBRARYDIR=G:/libs/boost_1_61_0/lib64-msvc-14.0
Hit configure and cmake should now be asking about Protobuf.
Hit configure again to get to the next set of variables that you need to set.
Hit configure again, note that for the library file, it is a list with the 'optimized' and 'debug' flag set before each variable.
And again for LevelDB
And for snappy:
For OpenCV set OpenCV_DIR to G:/libs/opencv
CUDNN
Download CUDNN5 from nvidia and extract the contents to G:/libs/cudnn5 so that the folder structure looks like:
G:/libs/cudnn5/bin
G:/libs/cudnn5/include
G:/libs/cudnn5/lib
Set CMake variables as follows:
CUDNN_INCLUDE=G:/libs/cudnn5/include
CUDNN_LIBRARY=G:/libs/cudnn5/lib/x64/cudnn.lib
Hit configure.
Set the BLAS option to Open
Hit configure again.
Set the following variables:
OpenBLAS_INCLUDE_DIR=G:/libs/caffe_deps/openblas/include
OpenBLAS_LIB=G:/libs/caffe_deps/openblas/lib/libopenblas.dll.a
********** IMPORTANT CUDA BUG ************************
CUDA_HOST_COMPILER=C:/Program Files (x86)/Microsoft Visual Studio 14.0/VC/bin/cl.exe
Hit configure, last time if everything is setup correctly.
For me, python automatically was configured: (Screenshot from a failed attempt with python 3.6)
And this is the config summary:
******************* Caffe Configuration Summary *******************
General:
Version : 1.0.0-rc3
Git : rc-1606-gec05d28-dirty
System : Windows
C++ compiler : C:/Program Files (x86)/Microsoft Visual Studio 14.0/VC/bin/x86_amd64/cl.exe
RelWithDebInfo : /MD /Zi /O2 /Ob1 /D NDEBUG /DWIN32 /D_WINDOWS /W3 /GR /EHsc /MP /MP
Release CXX flags : /MD /O2 /Ob2 /D NDEBUG /Oy- /Zo /Oy- /DWIN32 /D_WINDOWS /W3 /GR /EHsc /MP /MP
Debug CXX flags : /D_DEBUG /MDd /Zi /Ob0 /Od /RTC1 /DWIN32 /D_WINDOWS /W3 /GR /EHsc /MP /MP
Build type : Release
BUILD_SHARED_LIBS : ON
BUILD_python : ON
BUILD_matlab :
BUILD_docs :
CPU_ONLY : OFF
USE_OPENCV : ON
USE_LEVELDB : ON
USE_LMDB : ON
ALLOW_LMDB_NOLOCK : OFF
Dependencies:
BLAS : Yes (Open)
Boost : Yes (ver. 1.61)
protobuf : Yes (ver. 2.6.1)
lmdb : Yes (ver. 0.9.14)
LevelDB : Yes (ver. 1.2)
Snappy : Yes (ver. 1.1.1)
OpenCV : Yes (ver. 3.1.0)
CUDA : Yes (ver. 8.0)
NVIDIA CUDA:
Target GPU(s) : Auto
GPU arch(s) : sm_20 sm_21 sm_30 sm_35 sm_50 sm_60
cuDNN : Yes (ver. 5.1.5)
Python:
Interpreter : C:/Python35/python.exe (ver. 3.5.2)
Libraries : optimized C:/Python35/libs/python35.lib debug C:/Python35/libs/python35_d.lib (ver 3.5.2)
NumPy : C:/Python35/lib/site-packages/numpy/core/include (ver 1.11.2rc1)
Install:
Install path : G:/libs/caffe
If everything looks good, hit generate and you should have your visual studio solution.
Open your solution and hit build all.